Introduction to A/B Testing
What is A/B Testing?
A/B testing, sometimes referred to as split testing, compares two versions of a webpage, email, or other marketing material to see which one works better. Marketers can optimize their digital marketing tactics by experimenting with different variables, such as headline, picture, or call-to-action (CTA), and evaluating the outcomes. This allows for data-driven decision-making.
Why A/B Testing is significant in Digital Marketing
Making smart choices is essential in the quick-paced world of digital marketing. A/B testing offers a methodical way to figure out what your audience responds to the most. It removes uncertainty, enabling marketers to build their plans on factual information. This optimizes ROI in addition to increasing marketing efforts’ efficacy.
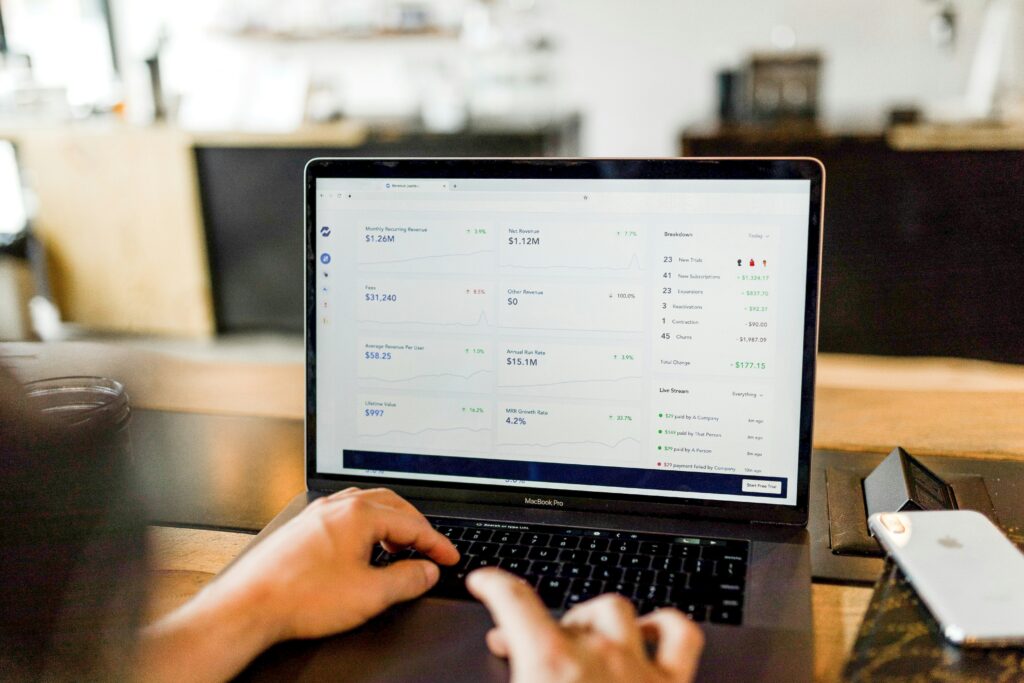
Advantages of A/B Testing in Digital Marketing
Boosting Conversion Rates
The power of A/B testing to raise conversion rates is one of its main advantages. Marketers may determine which components of a landing page, email, or advertisement result in the highest conversion rates by methodically testing many iterations of the content. For instance, altering a CTA button’s color can have a big impact on how many users finish a desired activity.
Improving the User Experience
A vital aspect for effective digital marketing is user experience, or UX. Marketers can test various layouts, design components, and content combinations using A/B testing to determine which combinations yield the greatest user experience. Higher engagement rates and more customer satisfaction follow from this.
Data-Driven Decision Making
A/B testing provides concrete data that helps in making good choices regarding informed marketing decisions. Marketers can use the findings of A/B tests to inform their strategy rather than depending solely on gut feeling or presumptions. This results in better resource allocation and campaigns that are more successful.
Reducing Risks and Uncertainty
It can be dangerous to implement adjustments to a digital marketing plan, particularly if those adjustments are predicated on conjecture. This risk is reduced with A/B testing, which shows what works and what doesn’t. This guarantees that marketing initiatives have a higher chance of success and lessens the uncertainty that comes with making changes.
How to Conduct A/B Testing
- Setting Clear Goals and Objectives
It’s critical to establish precise goals and objectives prior to initiating an A/B test. What are your objectives for taking this test? Are you trying to boost user engagement, improve conversion rates, or raise click-through rates? The entire testing process will be guided by a well-defined aim, which will also enable you to monitor success precisely.
- Identifying Relevant Variables to Test
A successful A/B test depends on choosing which variables to test. Typical variables consist of headlines, pictures, calls to action, forms, and general design. To make sure you can precisely ascertain how each element affects performance, concentrate on just one at a time.
- Creating Variants for Tests
Make two versions of your marketing asset: Version A (the control) and Version B (the variation) after you’ve determined which variable to test. Make sure the variable you are testing is the only thing that differs between the two versions. It is essential to isolate the variables in order to get precise results.
- Implementing the Test
To conduct the A/B test, divide your audience into two groups at random. Version A will be shown to one group and Version B to the other. To control this procedure and guarantee that the test is carried out impartially, use an A/B testing tool.
- Evaluating the Outcomes
Examine the test’s outcomes when it has been running for the proper amount of time. Examine important indicators including engagement levels, click-through rates, and conversion rates. Calculate the difference in performance between the two versions. Make sure the results are significant and not the result of chance by using statistical analysis.
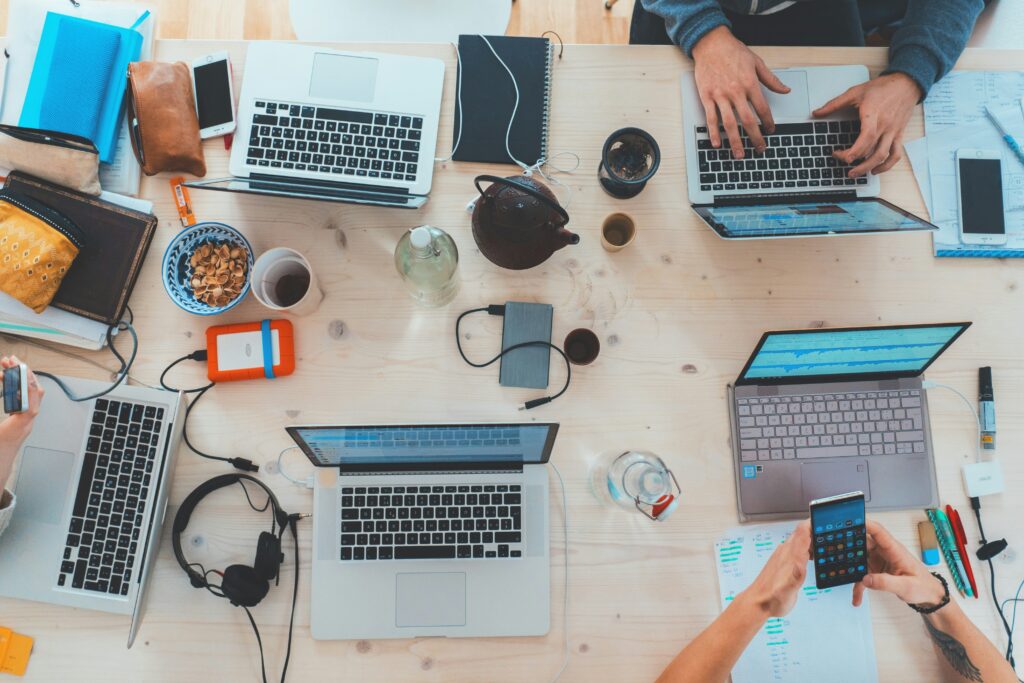
Top Strategies for A/B Testing
- Examining One Factor at a Time
Test just one variable at a time to guarantee accurate and useful results. This enables you to pinpoint the influence of that variable and make accurate modifications in response to the outcomes.
- Ensuring Statistical Significance
Your test results need to be statistically significant in order to be considered dependable. This indicates that chance is not the cause of the performance difference between the two versions. To determine significance and make sure your sample size is sufficient, use statistical techniques.
- Conducting Tests for the Adequate Period of Time
The length of your A/B test is essential to getting precise findings. Too little time spent on a test could result in insufficient data collection, while too much time spent on it could cause results that are biased by other influences. Choose a timeframe that strikes a balance between gathering data and outside factors.
- Using Reliable Tools for A/B Testing
Several technologies can be used to efficiently manage and evaluate A/B testing. Several well-liked choices consist of VWO, Optimizely, and Google Optimize. These tools make it simpler to run and comprehend A/B testing by providing features like statistical analysis, performance tracking, and audience segmentation.
Avoid These Common Mistakes in A/B Testing
- Testing Too Many Variables at Once
Confusion can arise from testing more than one variable at once, making it challenging to identify the changes that are driving the observed differences in performance. To keep things clear, limit your testing to one variable at a time.
- Neglecting Sample Size
Results from a tiny sample size may not be trustworthy. Make sure there are enough test takers for the results to be statistically significant. This could mean extending the test’s duration or expanding its target population.
- Terminating Experiments Too Soon
Premature test termination might lead to missing data and incorrect conclusions. Let the test run through to the end to collect enough data and guarantee accurate results.
- Not Iterating Based on Results
The process of A/B testing never ends. After you’ve found a winning alternative, apply the knowledge you’ve obtained to further refine the design. Iterate and test new versions often to ensure that your marketing campaigns are always optimized.
Case Studies: Effective A/B Testing in Digital Marketing
Example 1: Landing Page Optimization
To improve conversion rates, a nearby e-commerce company ran an A/B test on their landing page. Two iterations of the page were evaluated, one including a conventional layout and the other with a more straightforward design that concentrated on a single CTA. Conversions increased by 20% as a result of the streamlined design, highlighting the effectiveness of precise and targeted messages.
Example 2: Enhancing the Effectiveness of Email Marketing
A software company aimed to increase email campaign open rates. One generic and one personalized subject line were evaluated. The importance of personalization in email marketing is demonstrated by the 15% increase in open rates that the personalized subject line generated.
Example 3: Improving Buttons with Calls to Action
On their price page, a SaaS company tried two different CTAs: “Start Your Free Trial” and “Get Started Today.” A 10% increase in click-through rates was seen for the “Start Your Free Trial” button, indicating that providing a risk-free trial may make a product more desirable to prospective buyers.
The Future of A/B Testing in Digital Marketing
Integrating machine learning with artificial intelligence
A/B testing is about to undergo a revolution thanks to machine learning and artificial intelligence (AI). Large volumes of data may be swiftly analyzed by these technologies, which can also spot patterns that human analysts might overlook. Additionally, AI can automate testing, which facilitates the execution of numerous tests concurrently and ongoing marketing strategy optimization.
Real-Time A/B Testing
Real-time A/B testing helps marketers to quickly modify their plans. Businesses can improve responsiveness and efficacy of their campaigns by making quick adjustments to them based on current results when they have real-time data analysis capabilities.
Personalization and A/B Testing
In digital marketing, personalization is becoming more and more significant. Subsequent A/B testing is probably going to concentrate more on tailored experiences, experimenting with different offers and content for different audience segments. By ensuring that marketing initiatives are customized to individual interests, this strategy boosts relevance and engagement.
Conclusion
One important weapon in the toolbox of a digital marketer is A/B testing. It makes data-driven decisions possible, raises conversion rates, improves user experience, and lowers the risks involved in modifying marketing tactics. Businesses may fully utilize A/B testing to optimize their digital marketing efforts and achieve higher success by adhering to recommended practices and avoiding common pitfalls.
Frequently Asked Questions (FAQs)
What are the Best Tools for A/B Testing?
For A/B testing, there are numerous options accessible, such as Optimizely, Unbounce, VWO, and Google Optimize. These programs provide a range of capabilities to facilitate the efficient management, execution, and analysis of A/B testing.
How Long Should an A/B Test Run?
The volume of traffic and the intended level of statistical significance determine how long an A/B test takes. Tests should often run for one to two weeks in order to collect enough data, though the precise length will depend on the objectives and size of the audience.
Is it Possible to Use A/B Testing for Mobile Apps?
The answer is yes, mobile apps can benefit from A/B testing. Businesses may enhance user experience and boost engagement and retention rates by experimenting with various app interfaces, features, and user flows. Mobile app testing is the focus of tools like Apptimize and Firebase A/B Testing.